Education
Take care of an early warning – Nursing Education Network
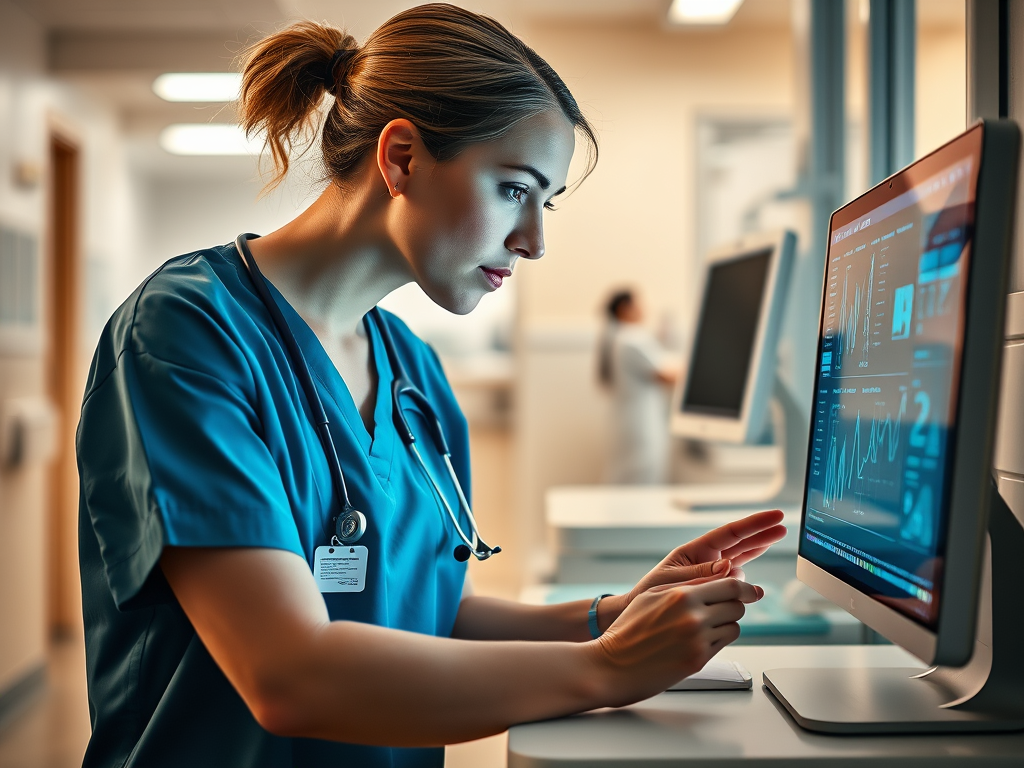
Ml Millenson. (2025). Ai uses what nurses see and the danger of death is 36% – however the grant pulled. Forbes magazine.
In this text, he emphasizes a major innovation in healthcare. The artificial intelligence system, communicating the narrative fears introduced within the early warning RN (EWS) system, analyzes the observations of nurses. The system provides for a deterioration of the patient. A study in 4 hospitals showed an unusual 36% reduction within the variety of deaths within the hospital using fears. There was also a 11.2% decrease in stay. AI’s ability to translate nurses’ insights into basic data allowed for earlier interventions. This ability reduced the danger of conditions comparable to sepsis.
This significantly affects the mortality of patients in hospital conditions. Uses patterns in nurses’ observations. He also uses nuances of their documentation in electronic health documentation (EHR).
Here is the division of how it really works:
- Analysis of nursing supervision patterns: Many traditional early warning systems are based totally on physiological data. They give attention to life parameters and laboratory results.
- EWS is targeted on the patterns of nurses’ supervision documentation. This includes an evaluation of metadata their EHR activities.
- Increased grade frequency: for instance, how often the nurse checks the patient’s respiratory rate.
- Assembly in unusual times: comparable to checking life parameters in the midst of the night in a patient from outside ICU.
- Interventions for administering nursing drugs: if not administering a planned medicine, since the patient is unstable.
- Turning on narrative fears: The system also uses natural language processing to discover mention of care within the narrative notes of nurses. This is captured by the qualitative elements of the nurses’ assessment, which is probably not present within the structured data.
- Identification of early deterioration: Patterns of increased supervision and documented care indicate the clinical intuition of a nurse. This intuition suggests that the patient’s condition is getting worse. Take care of the processing of this data through the machine learning algorithm. It can discover patients prone to worsening as much as 42 hours sooner than models based on physiological indicators.
- Ensuring practical information: The system calculates the results of the danger of decay. This result may be green, yellow or red. It is exhibited to the EHR care team. This provides a selected data point. May result in motion. This happens even when the care of a nurse may be more “feeling”. The system also offers an in depth division of risk assessment. It includes contributing aspects. Trends in time are also shown. This increases transparency and explanation.
- Facilitating timely interventions: earlier prediction of decay gives clinicians a greater time of implementation for the implementation of obligatory interventions. This may include closer monitoring and changes in treatment. The results of the study showed an increased speed of unexpected transfers of the Intensive Care Department (ICU). The study showed a rise of 24.9% of the immediate risk of an unexpected ICU transfer within the intervention group.
- Improving patients’ results: enabling prior interventions, it has been shown that EWS has a major positive impact on patient results. The study showed a 35.6% decrease within the immediate risk of mortality within the hospital in patients monitored by the AI system in comparison with atypical care. In the intervention group there was also 7.5% reduced immediate risk of sepsis.
Ews worries like a “second pair of eyes”. He uses expert knowledge and observations of nurses in real time. This helps to discover subtle signs of decay that traditional systems can miss. The care team detects problems early. Their timely activities are key aspects in a major reduction within the mortality rates of patients observed within the study.
Rossetti, SC, Dykes, PC, Knaplund, C. and others Real time supervision system for worsening patients: pragmatic controlled cluster test. Night with (2025).
Rossetti, S. et al. Modeling the healthcare process for clinotypical behavior to make use of the profit of a specialized specialist signal (HPM – Expertsignals): Development and evaluation of conceptual frames 0. J. Am. Med. Inform. Assoc. 1242–1251 (2021).
-
Global Health4 months ago
The Global Fund opens up the potential of private sector investment – updates
-
Well-Being4 months ago
Fast and healthy advice on preparing meals for busy nurses
-
Well-Being4 months ago
5 books that may help at work at work
-
Best Practice5 months ago
A cultural approach to the treatment of neonatal pain
-
Well-Being3 months ago
Maintenance of the nursing engine – each day nurse
-
Education4 months ago
Including diversity within the expression of English
-
Well-Being3 months ago
How to get the standard of sleep for higher mental health
-
Global Health5 months ago
Global Fund establishes pioneering climate and health fund to speed up global motion – press releases